Optimizing patient outcomes through healthcare analytics
Determine where to distribute medical staff based on patient vulnerability and past morbidity
Identify populations most vulnerable to flu morbidity and mortality
Determine which U.S. states have the highest need for medical staff during the upcoming flu season
Develop data-backed recommendations on where to send care staff
Data
Context
TopMed Healthcare Staffing has a limited number of available personnel and a nationwide network of hospitals. They needed to know which states would need the most [patient care in the upcoming flu season.
Professional Competencies
Data profiling
Data cleaning
Data transformation & integration
Statistical analysis
Hypothesis testing
Statistical & temporal visualizations
Spatial analysis
Storytelling with data
Objectives
This project utilizes two data sets:
Influenza death statistics from the Centers for Disease Control and Prevention (CDC)
Population data from the U.S. Census Bureau
Phase 1: Identifying Vulnerable Populations
TopMed Healthcare Staffing started with the assumption that young children and elders were more vulnerable to serious illness from influenza, but early analysis showed a much stronger correlation between advanced age and flu mortality.
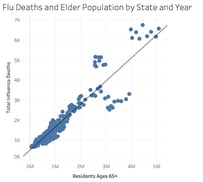
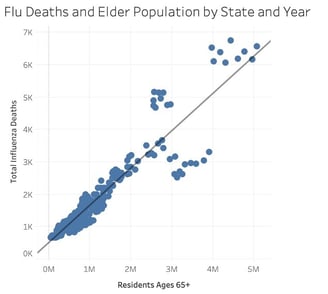
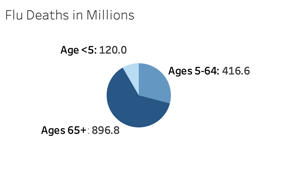
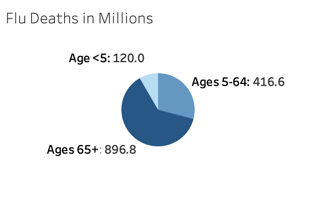
Phase 2: Run Temporal Analysis to Define Flu Season
TopMed needed a time frame for flu season to plan staff assignments. I hypothesized that colder regions would have a dramatically higher increase in flu deaths during the winter, while warmer regions had less variability. The data disproved that hypothesis, indicating that all regions had a spike from November to April. Our strategy would not be able to rely on shifting staff into cold-weather regions during that peak season.
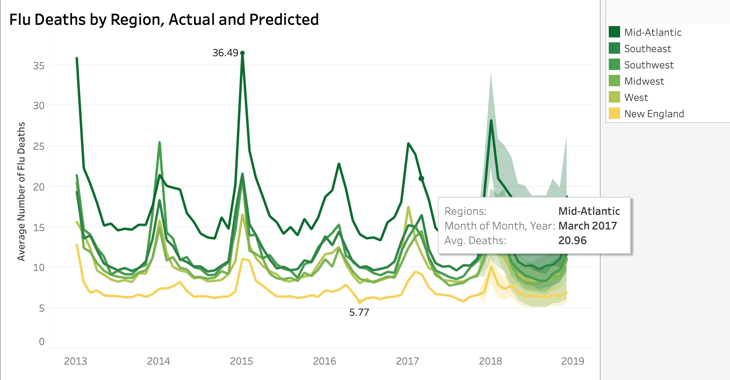
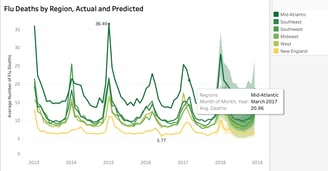
Phase 3: Identify Highest-Need Regions
Having learned that older adults were the most likely to have severe flu outcomes, I used that information to identify the states needing more hospital patient care staff. I chose to analyze this information across two dimensions: the total number of adults 65+ and the proportion of these older adults to the total population.
I discovered that a high count of older adults did not always correlate with a high proportion. I realized that states in both categories would have to be "high-need" in terms of staffing.
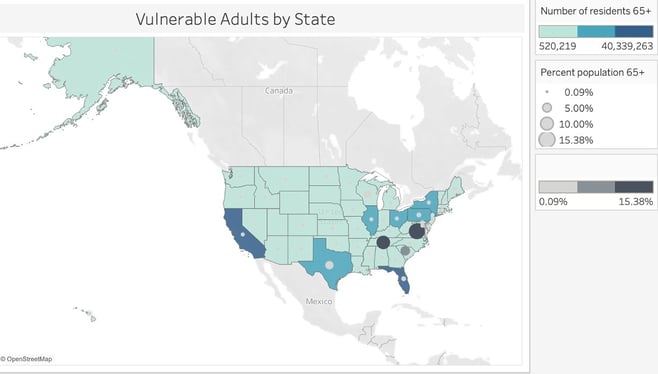
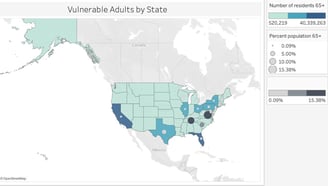
Phase 4: Develop Actionable Categorization System
I had drawn two conclusions. First, we would primarily need to base our staff allocation strategies on the location of vulnerable elders, not on regional differences in flu season. Second, we needed to prioritize states with high numbers of elders as well as those with low numbers but high proportions of that vulnerable population.
I developed a three-tier staffing plan that categorized states into low, medium, and high needs. Each state would receive additional staff from November to April based on its level and total expected patient count, to be calculated via further analysis.
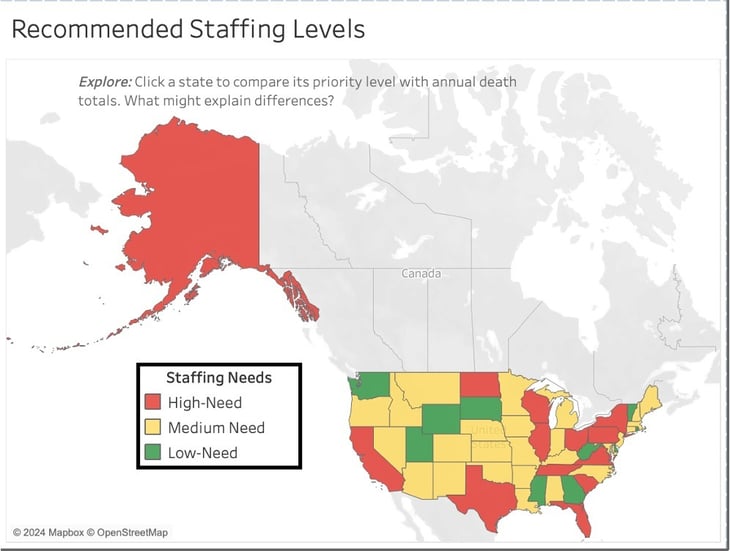
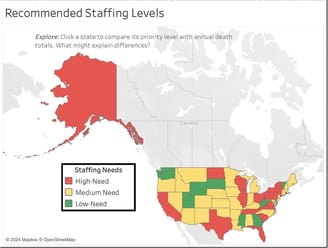
Recommendations for Further Analysis
How have needs changed in recent years? The data available to us only extends to 2016. Healthcare staffing strategies and patient needs have shifted significantly since the COVID pandemic.
Where does TopMed have flexibility in staffing outside of flu season? Given that resources are not available to hire temporary staff, we need to identify areas in which we can "borrow" staff for flu season needs during peak months.